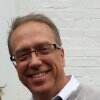
Is Self-Service Analytics Sustainable?
Self-service analytics are increasingly being implemented by organizations that want to promote a data-driven culture. But how sustainable is it? Read more.

What is Self-Service Analytics?
Self-service analytics is a common buzzword for many organizations which desire to be more data driven and less dependent on IT for their data needs. Organizations are increasingly implementing self-service capabilities to enable and promote a data-driven culture within their organizations. Gartner, Inc. predicts that by 2019, the analytics output of business users with self-service capabilities will surpass that of professional data scientists. Self-service analytics sound great, but how sustainable is it? In a series of articles, we will dive into self-service, starting first with “What is self-service analytics?”.Every user wants to be self-service enabled, but not all users have the same skillset. Therefore, enabling self-service means different things to different users. Let’s first start by defining some basic user personas, their skillsets and what self-service means to each of them.
- General Consumer - Analytic users who leverage reports and dashboards from trusted, governed data sources to run the day-to-day business. They work exclusively from an operational perspective. They leverage BI tools and dashboards to monitor and analyze the data.
- Data Analyst – Analytic users who run reports, manipulate or pivot on prepared data products. Access rules are usually restricted to prepared data products from trusted, governed data sources, which may be optimized structures, sometimes referencing common summaries. They primarily work from an operational perspective but do some data discovery as well, when needed. They may leverage the same BI tools as the general consumer but are also savvy at writing SQL code. They can directly query data which has been curated to a tabular structure, e.g. a relational database schema, or a schema defined by Hive or some other interface that enables data queries. They are skilled spreadsheet users.
- Citizen Data Scientist - Data analyst which can query a broad range of data products looking for answers to new business problems or discover new insights from existing data which is trusted and governed. They may also leverage new experimental data. They work primarily from an R&D perspective. These individuals typically have a strong, business subject area knowledge, SQL coding skills and an understanding of how to apply statistical and machine learning algorithms as a black box in solving business problems.
- Data Scientist - Work with any data source (raw or curated) to look for answers to new business problems or discover new insights. They will bring their own data and augment it with existing data as needed. They most often work from a R&D perspective. They have the most diverse skill set, e.g. strong interpretive language skills (e.g. Python, R) and SQL skills, and solid mathematic skills for leveraging machine and deep learning algorithms (often a Ph.D. in science or mathematics). They might be a business subject matter expert or work with a business subject matter expert to better understand the business problem they are trying to solve.
General consumers reach into the Access Layer (typically star schemas) via OLAP tools and dashboards. Data analysts leverage the Access Layer and reach further into the Integration Layer (typically a near 3rd normal form structure) to do ad-hoc analytics. Citizen data scientists leverage both the Access and Integration Layers, but also leverage standardized data (typically flattened structures, but not necessarily) in the Acquisition Layer. The data scientists, with their unique skillset, have full access to all forms of data within the entire analytics ecosystem. All users will leverage trusted and governed data, where possible, as it possesses the highest data quality and requires the least effort to utilize.
All personas, except the general consumers, need to provision resources for their self-service analytics. These terms can mean something different to each persona and are described below to provide a more consistent definition in support of managing self-service analytics.
Automated-Provisioning: The goal should be to enable users to request resources which can be systematically enabled within sanctioned areas, if they fall within standard defaults, e.g. storage, compute, memory. An exploration zone, such as Teradata Data Labs, is a good example of this capability in action.
Self-Service Analytics: The ability for the user to access and utilize available resources (e.g. storage, compute, memory) so that they can acquire, profile, wrangle and analyze data (structured or unstructured) for some analytical purpose on their own. This could be a data scientist doing advanced analytics or a data analyst running SQL and NoSQL queries or connecting an OLAP tool for investigating new reports and dashboards. They may or may not require new data. By far the largest segment of self-service analytics are the general consumers, leveraging BI tools to slice and dice within the tool’s defined data domain.
Individuals doing self-service analytics should be free to do what they need by themselves. They read, seek out online training, attend instructor led training classes, etc. They learn as they go, most often when they need to remove a roadblock. Publishing and maintaining standards and best practices further enhances self-paced learning.
Some users will need to load data into a relational database, which means writing DDL code, either by using command line or GUI tools. A data analyst is very familiar with SQL, so creating a table and loading it should not be a stretch. A citizen data scientist may also be able to load data into NoSQL structures and perhaps wrangle and conform some data into a usable format. The data scientist can do pretty much everything needed to load and prepare any type of data.
At some point, exploration results and model definition techniques that both lead to reuse and faster time-to-value are important enough to be recalculated and reanalyzed on a regular basis and should become part of the organization’s critical production processing. Operationalization is the process by which data and/or analytic modules are moved from an exploration environment into a production environment. It entails adhering to data naming conventions, optimizing processes and utilizing standard services, thereby ensuring the data and analytics will be consistently available to the entire user community. Perhaps even more important, it frees up users to focus on other research needs.
Everyone wants to speed up self-service analytics. Depending on the choices made during exploration, the operationalization effort may take longer. A common example would be using tools for exploration, which are not authorized in production. The more aware the users are, the better they understand the trade-offs.
There is a lot of hype around self-service analytics. The complexity goes up when a user decides to bring their own data; therefore, making data easier to find and access will greatly accelerate self-service analytics initiatives and minimize data sprawl.
In part 2 of this series we will discuss the value and challenges of self-service analytics within organizations today.
알고 있어
테라데이트의 블로그를 구독하여 주간 통찰력을 얻을 수 있습니다